Table of Contents
Introduction
The world of AI is moving fast, with breakthroughs and improvements coming monthly, weekly, and, at times, even daily. Stable Diffusion (most commonly used to generate images based on text prompts) has seen especially rapid growth recently, with new interfaces springing up faster than most people can keep up with. In addition to these 3rd party packages designed to make Stable Diffusion accessible to the masses, GPU manufacturers (and others) are also making significant contributions to provide massive leaps in performance.
In fact, in the last few weeks, both NVIDIA and AMD have put out information on achieving the best performance currently possible for one of the most common implementations of Stable Diffusion: Automatic 1111.
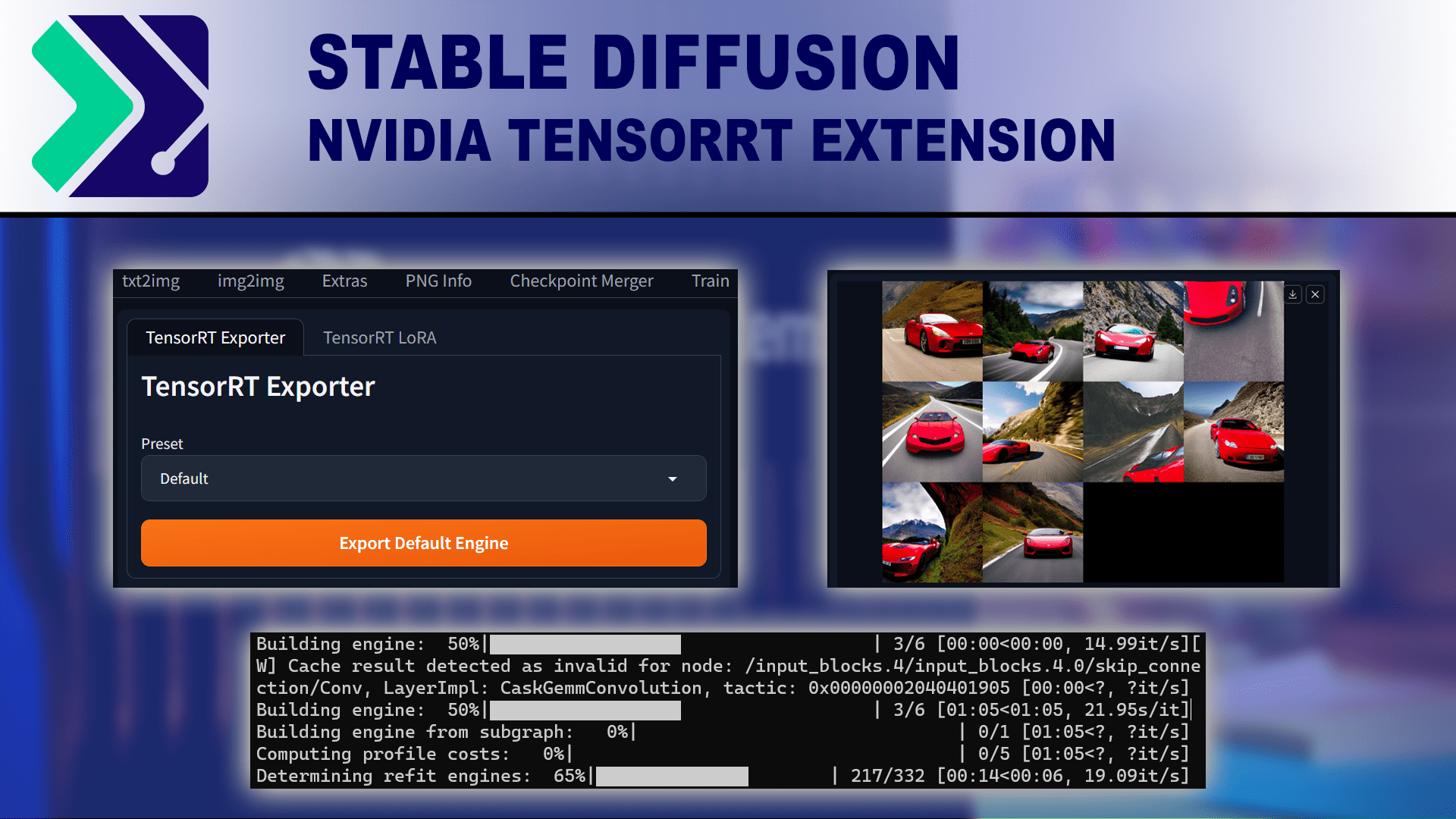
In this article, we want to focus on a recently released TensoRT extension from NVIDIA, which they say should improve performance by almost 3x over the base installation of Automatic 1111, or around 2x faster than using xFormers. This extension creates optimized engines for your specific GPU, and is fairly easy to install and use. All told, following their guide took us about 15 minutes from start to finish and would be even faster if you already have a fully updated version of Automatic 1111 on your system. For more details on how it works and how to use it, we recommend reading NVIDIA’s post: TensorRT Extension for Stable Diffusion Web UI.
Similarly, AMD also has documentation on how to leverage Microsoft Olive ([UPDATED HOW-TO] Running Optimized Automatic1111 Stable Diffusion WebUI on AMD GPUs) to generate optimized models for AMD GPUs, which they claim improves performance on AMD GPUs by up to 9.9x. That is a huge performance uplift if true, and we will be looking at that in a sister article that you can read here: AMD Microsoft Olive Optimizations for Stable Diffusion Performance Analysis.
While we are primarily looking at the NVIDIA TensorRT extension in this post, we will quickly compare NVIDIA and AMD GPUs at the end of this article using both optimizations to see how each brand compares after everything is said and done.
Test Setup
Threadripper PRO Test Platform
CPU: AMD Threadripper PRO 5975WX 32-Core |
CPU Cooler: Noctua NH-U14S TR4-SP3 (AMD TR4) |
Motherboard: ASUS Pro WS WRX80E-SAGE SE WIFI BIOS Version: 1201 |
RAM: 8x Micron DDR4-3200 16GB ECC Reg. (128GB total) |
GPUs: NVIDIA GeForce RTX 4090 24GB NVIDIA GeForce RTX 4080 16GB Driver Version: Studio 537.58 |
PSU: Super Flower LEADEX Platinum 1600W |
Storage: Samsung 980 Pro 2TB |
OS: Windows 11 Pro 64-bit (22621) |
AMD Ryzen Test Platform
CPU: AMD Ryzen 9 7950X |
CPU Cooler: Noctua NH-U12A |
Motherboard: ASUS ProArt X670E-Creator WiFi BIOS Version: 1602 |
RAM: 2x DDR5-5600 32GB (64GB total) |
GPUs: NVIDIA GeForce RTX 4090 24GB NVIDIA GeForce RTX 4080 16GB Driver Version: Studio 537.58 |
PSU: Super Flower LEADEX Platinum 1600W |
Storage: Samsung 980 Pro 2TB |
OS: Windows 11 Pro 64-bit (22621) |
Benchmark Software
Automatic 1111 v1.6 Python: 3.10.6 xFormers: 0.0.20 SD Model: v1.5 |
TensorRT Extension for Stable Diffusion Web UI |
We originally intended to test using a single base platform built around the AMD Threadripper PRO 5975WX, but through the course of verifying our results against those in NVIDIA’s blog post, we discovered that the Threadripper PRO platform could sometimes give lower performance than a consumer-based platform like AMD Ryzen or Intel Core. However, Intel Core and AMD Ryzen tended to be within a few percent, so, for testing purposes, it doesn’t matter if we used an AMD Ryzen or Intel Core CPU.
Since it is common for Stable Diffusion to be used with either class of processor, we opted to go ahead and do our full testing on both a consumer- and professional-class platform. We will largely focus on the AMD Ryzen platform results since they tend to be a bit higher, but we will also include and analyze the results on Threadripper PRO.
As noted in the Introduction section, we will be looking specifically at Automatic 1111 and will be testing it in three configurations: a base install, with xFormers enabled, and with the new TensorRT extension from NVIDIA. Since we need to manually re-generate the engine each time we change the GPU, our testing is significantly more manual than we normally prefer. Because of this, we opted to test with just two GPUs to see how the performance gains change depending on the card. For now, we chose the NVIDIA GeForce RTX 4080 and the RTX 4090, but pro-level cards like the RTX 6000 Ada should show similar performance uplifts.
For the specific prompts and settings we chose to use, we changed things up from our previous Stable Diffusion testing. While working with Stable Diffusion, we have found that few settings affect raw performance. For example, the prompt itself, CFG scale, and seed have no measurable impact. The resolution does, although since many models are optimized for 512×512, going outside of that sometimes has unintended consequences that are not great from a benchmarking standpoint. We do want to test 768×768 with models made for that resolution in the future, however.
To examine this TensorRT extension, we decided to set up our tests to cover four common situations:
- Generating a single high-quality image
- Generating a large number of medium-quality images
- Generating four high-quality images with minimal VRAM usage
- Generating four high-quality images with maximum speed
To accomplish this, we used the following prompts and settings for txt2img:
Prompt: red sports car, (centered), driving, ((angled shot)), full car, wide angle, mountain road
txt2img Settings | Test 1 | Test 2 | Test 3 | Test 4 |
---|---|---|---|---|
Steps | 150 | 50 | 150 | 150 |
Width | 512 | 512 | 512 | 512 |
Height | 512 | 512 | 512 | 512 |
Batch Count | 1 | 10 | 4 | 1 |
Batch Size | 1 | 1 | 1 | 4 |
CFG Scale | 7 | 7 | 7 | 7 |
Seed | 3936349264 | 3936349264 | 3936349264 | 3936349264 |
We will note that we also tested using img2img, but the results were identical to txt2img. For the sake of simplicity, we will only include the txt2img results, but know that they also hold true when using an image as the base input as well.
NVIDIA GeForce RTX 4090 Performance Gains
Starting with the NVIDIA GeForce RTX 4090 24GB, you may first notice that many of our testing prompts seem a bit redundant. As long as we kept the batch size to 1 (the number of images being generated in parallel), the iterations per second (it/s) are pretty much unchanged with each of the three methods we looked at (base, xFormers, and TensorRT extension). This is expected behavior since this metric essentially reports how many “steps” we can complete each second. In more standard GPU performance testing, we would likely do one of these three tests and call it a day, but we wanted to check to ensure there were no gaps with the TensorRT extension. If it turned out to only work with a batch count of 1, or only up to a certain number of steps, that is very important information to have.
As far as the performance gains go, with the RTX 4090 on the AMD Ryzen platform, the TensorRT extension gave us about a 2.3x increase in performance over a basic Automatic 1111 installation, or about a 1.7x increase compared to a setup utilizing xFormers.
This is a bit less than what NVIDIA shows on their TensorRT Extension for Stable Diffusion Web UI blog post, but they don’t list the base platform, so it may be due to system configuration differences. In fact, when we did the same testing on an AMD Threadripper PRO platform, the TensorRT extension gave us a much larger performance gain – about a 3.4x increase in performance over a basic Automatic 1111 installation, or about a 2.6x increase compared to a setup utilizing xFormers when generating images in serial. Generating multiple images in parallel (with a batch size of 4) showed a little smaller gain of 2.3x and 1.5x compared to stock and xFormers, respectively.
Interestingly, the difference in speedup between the two base platforms is not due to the TensorRT extension – both the Ryzen and Threadripper PRO platforms gave almost identical results with the RTX 4090 when using the new extension. The reason the Ryzen platform shows a smaller benefit is because it is faster when using the base install of Automatic 1111, or when enabling xFormers, so the relative performance gain is smaller.
No matter how you slice it, this TensorRT extension can greatly increase performance and make the extra ~15 minutes of set-up time well worth it if you plan to use Automatic 1111 to generate a decent number of images. Across both platforms, this averages out to about a 2.75x increase in performance over a basic Automatic 1111 installation, or about a 2x increase compared to xFormers.
NVIDIA GeForce RTX 4080 Performance Gains
Moving on to the NVIDIA GeForce RTX 4080 12GB, the performance gains are still very impressive, although slightly less than with the more powerful RTX 4090. With the RTX 4080 on a Ryzen platform, we saw about a 2.5x speedup with the TensorRT extension compared to a basic Automatic 1111, or about a 1.75x improvement compared to using xFormers.
Once again, the performance gains when running on a Threadripper PRO platform are a bit higher, mostly because that platform gets lower results with the base Automatic 1111 install and xFormers. Because of this, when using the RTX 4080 on Threadripper PRO, we saw about a 2.8x speedup with the TensorRT extension compared to a basic Automatic 1111, or about a 2.1x improvement compared to using xFormers. Once again, the gain is slightly less when using a larger batch size, this time to the tune of 2.4x and 1.6x compared to stock and xFormers, respectively.
Across both platforms, this averages out to about a 2.6x increase in performance over a basic Automatic 1111 installation, or about a 1.9x increase compared to xFormers.
RTX 4080 vs RTX 4090 vs Radeon 7900 XTX for Stable Diffusion
While a performance improvement of around 2x over xFormers is a massive accomplishment that will benefit a huge number of users, the fact that AMD also put out a guide showing how to increase performance on AMD GPUs by ~9x raises the question of whether NVIDIA still has a performance lead for Stable Diffusion, or if AMD’s massive performance gain is enough for them to take the lead.
To show how NVIDIA and AMD stack up right now, we decided to take the results from the “Batch Size: 4” and “Batch Count: 4” tests in this article, as well as our AMD-focused article looking at a similar performance optimization guide recently published by AMD, to show how the RTX 4090, RTX 4080, and Radeon 7900 XTX stack up. We will note that lower-end cards may show different relative performance between each brand, but this gives us a good look at how the performance compares at the top end:
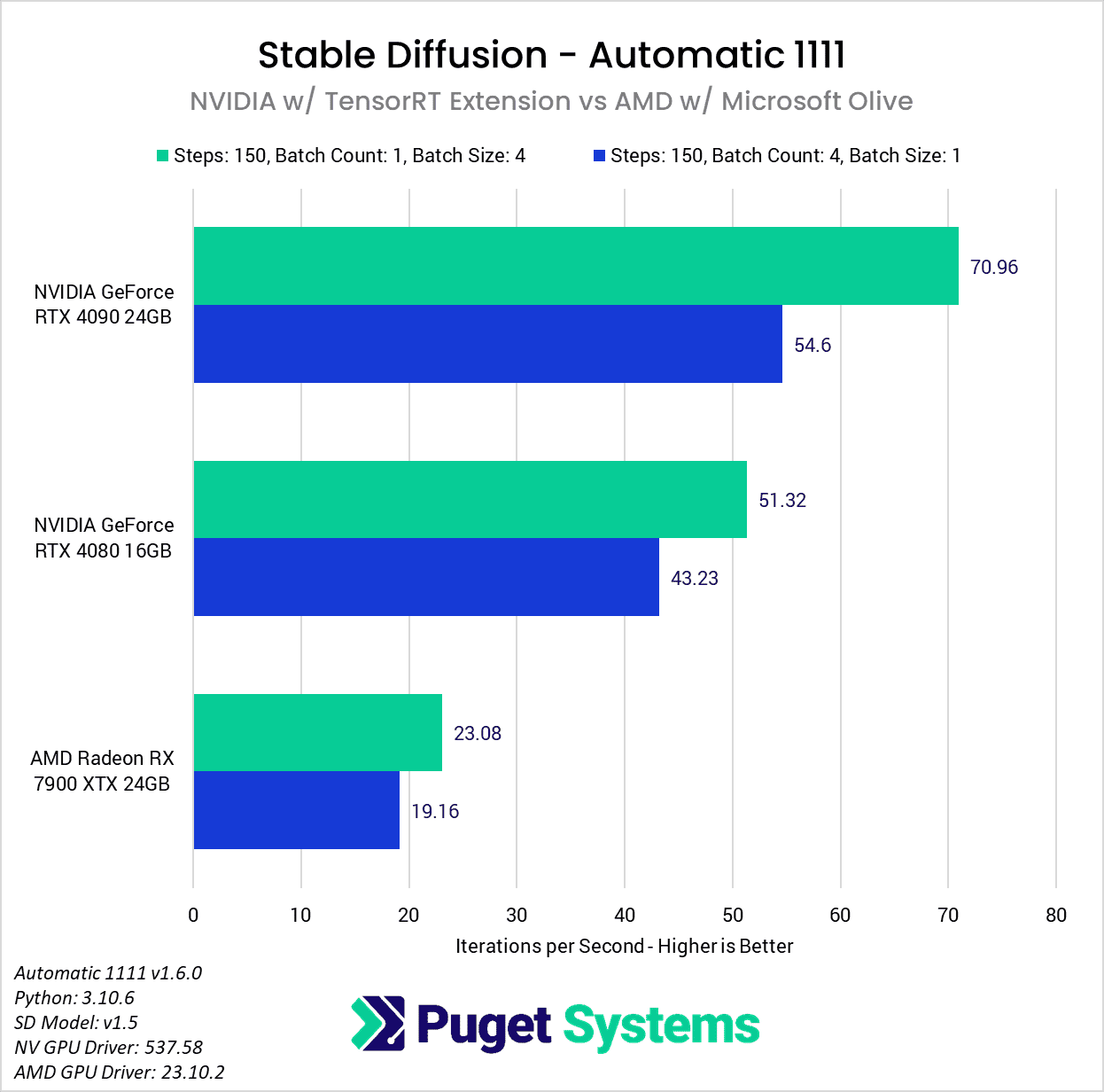
Unfortunately for AMD, even with the huge performance gains we saw in Automatic 1111 (averaging around 11x faster than a base A1111 DirecML install), it isn’t enough to bring the Radeon 7900 XTX anywhere close to the RTX 4080. It does bring Automatic 1111 on AMD to within a few it/s of what we see in other SD implementations like SHARK, but that only gets the 7900 XTX to be close to the performance of an RTX 4080 using xFormers (23 vs 24 it/s). With the TensorRT extension, NVIDIA again catapults themselves into a strong lead.
The 7900 XTX does have more VRAM than the RTX 4080 (24GB vs 16GB), but that will most often only affect how many images you can generate in parallel, and the 16GB on the RTX 4080 is already enough to generate eight images at once (the max allowed in Automatic 1111) at 512×512 resolution. Even if you are working at large enough resolutions for the VRAM difference to come into play, it doesn’t make any sense to use a 7900 XTX to get the ~20% performance bump from a larger batch size, when using an RTX 4080 can be almost 2x faster even when limited to generating images in serial.
All told, with the current optimizations recommended by both NVIDIA and AMD, we are looking at about 2.2x higher performance with an NVIDIA GeForce RTX 4080 over the AMD Radeon 7900 XTX, or 3x higher performance with a more expensive RTX 4090.
Conclusion
Artificial Intelligence and Machine Learning is both a fun and frustrating field to perform this type of testing for because it changes and evolves extremely quickly. This means that there are always new and interesting things to examine, but also that any performance data becomes outdated faster than we can sometimes keep up with.
In our opinion, NVIDIA’s TensorRT extension for Automatic 1111 is very much in the “fun” category, simply because we can’t help but smile when 10-15 minutes spent following an optimization guide can net you a 2x or more performance gain. For a bit of context, with this extension, we could generate ten 512×512 images with 50 steps in about 10 seconds with an RTX 4090, or about an image every second! Even with the great work AMD has also done recently to improve Stable Diffusion performance for their GPUs, this currently cements NVIDIA as the GPU brand of choice for this type of work.
As we noted throughout this article, the exact performance gain you may see with this extension will depend on your GPU, base platform, and the settings you use in Automatic 1111. No matter how you slice it, if you run Stable Diffusion on an NVIDIA GPU this extension is definitely something you should check out. On average, the overall performance improvements we saw were:
- 2.7x faster than a base Automatic 1111 install
- 1.9x faster than Automatic 1111 with xFormers
If you use Stable Diffusion on an NVIDIA GPU, we would be very interested to hear about your experience with the TensorRT extension in the comments! And if you are looking for a workstation for any Content Creation workflow, you can visit our solutions pages to view our recommended workstations for various software packages, our custom configuration page, or contact one of our technology consultants for help configuring a workstation that meets the specific needs of your unique workflow.